Wovenware lands federal patent for synthetic data generation method
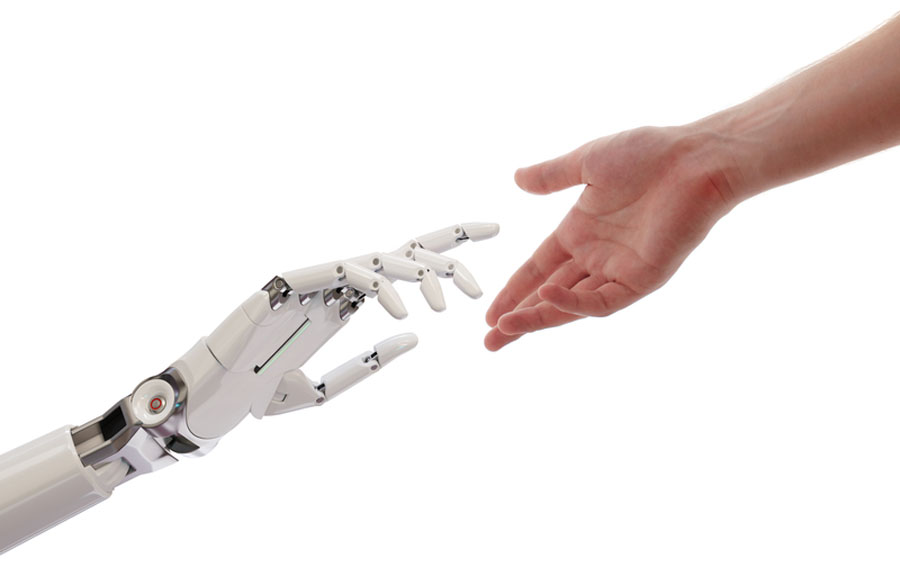
Wovenware, a nearshore provider of Artificial Intelligence (AI) and digital transformation solutions, announced that the US Patent and Trademark Office has issued it Patent No. 11,024,032 B1.
The patent addresses the company’s system and method for generating synthetic overhead imagery based on cluster sampling and spatial aggregation factors of existing data-sets.
Wovenware uses the patented method to “develop highly accurate deep learning-based computer vision models” for government and commercial applications in regulated industries.
“The training of deep learning models using large amounts of annotated data has become essential to the accuracy of computer vision applications,” said Wovenware CEO Christian González.
“The issuance of this new patent recognizes Wovenware’s unique approach to generating synthetic datasets and reinforces our expertise in developing highly accurate solutions that enable automated object detection, such as satellite imagery or molecular object identification, within public and private sectors,” he said.
The newly issued patent protects Wovenware’s mechanisms and processes for enhancing limited data-sets by generating synthetic overhead imagery based on cluster sampling and spatial aggregator factors (SAFs).
It generates synthetic images through its unique process of cropping objects from original images and inserting them into uniform, natural or synthetic backgrounds. The objects are selected by clusters based on pixel distribution similarity and through SAF mining.
In 2020, Wovenware was listed as a Strong Performer in the Forrester New WaveTM: Computer Vision Consultancies report of the top 13 computer vision providers, joining companies such as Accenture, Capgemini, Deloitte and PwC.
Wovenware’s computer vision services are designed to help organizations optimize their operations by extracting valuable insights from the physical world.
When images are limited, Wovenware customizes algorithms to generate synthetic images with varying textures, backgrounds and other conditions, to complement actual images and create a diverse and large enough dataset to train a high-performing computer vision model.